Important steps in this process include data transformation, modeling, inspection, and sanitization. The primary goals of data analysis is to find relevant information, support conclusions, and ease the process of making decisions. It includes a wide range of approaches that can be tailored to different situations. Data analysis is being used in many different fields, including business, research, and social science. It supports more rational and fact-based decision-making processes, which promotes operational effectiveness and efficiency.
Primary and secondary data are the two main categories into which data is usually divided. Primary data, often called raw data, wherein the information gathered directly from the source by the researcher. On the other hand, secondary data originates from outside sources, including records kept by organizations or government agencies, or data that was first gathered for different types of research. These are very important characteristics in the field of social science research.
In order to guarantee accuracy & insightful interpretation, there are various procedures involved in survey data analysis for research purposes. Here’s a broad overview:
Data Preparation and Cleaning:
- Eliminate any answers that are duplicated or incomplete.
- Examine the data for anomalies or mistakes, then determine whether to eliminate or fix them.
- Transform data into a standardized format (numerical, categorized, etc.).
Descriptive Analysis:
- For numerical variables, compute basic statistics including mean, median, mode, standard deviation, and range.
- Determine the frequencies and proportions for each category when dealing with categorical variables.
- To comprehend the distribution of replies, visualize the data using pie charts, bar charts, histograms, and other visualization tools.
Exploratory Data Analysis (EDA):
- Explore relationships between variables using correlation coefficients for numerical variables and contingency tables for categorical variables.
- Use graphical tools like scatter plots, box plots, and heatmaps to visualize relationships.
Inferential Statistics:
- Conduct hypothesis tests to make inferences about the population based on the sample or primary data. Common tests include for analysis would be t-tests, ANOVA, chi-square tests, etc.
- Calculate confidence intervals at 95% confidence level to estimate the range within which population parameters lie.
Advanced Interpretation:
- To find correlations between dependent and independent variables, use regression analysis.
- For more intricate data exploration, apply methods such as factor analysis or cluster analysis.
Reporting and Interpretation:
- Interpret the results in the context of the research questions or hypotheses.
- Discuss the implications of the findings and any limitations of the study.
- Present the results clearly using tables, figures, and narrative descriptions
Overall, it is crucial for students to understand how to use statistical methods to examine survey data. These analyses can be carried out with the help of numerous statistical software programmes, including R, SPSS, AMOS-SEM and PLS-SEM. Further help can also be obtained by asking teachers or consultants for advice or by reviewing statistics-related literature and resources.

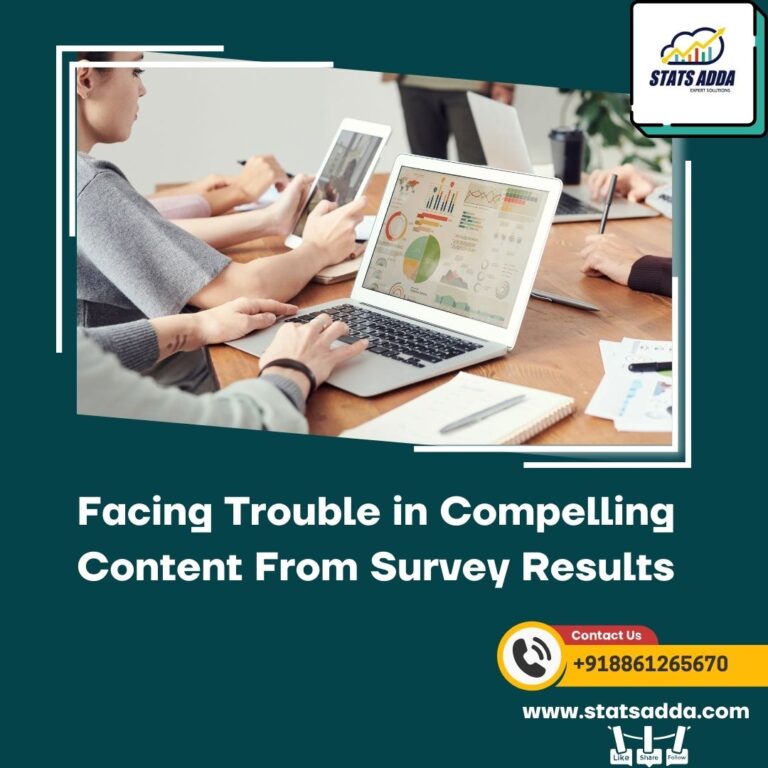
Here, in Stats Adda, we offer comprehensive support including data analysis for Phd. and management students by using SPSS & AMOS, implementation of PhD thesis work, consultancy services for Phd. management students, dissertation statistics help, statistical analysis services and many more. As a team, we ensure timely delivery, work accuracy, clear communication, and one month of post-completion support. Choose us as your partner in your data analytics journey.
Commonly Asked Questions
To select the appropriate statistical method for your survey data analysis, you should consider the following steps:
- Review your research questions and hypotheses
- Understand the appropriate variable type such as (nominal, ordinal, interval, or ratio) required for the survey data analysis.
- Determine the number of variables required.
- Then determine the type of data (Parametric or Non-parametric)
- Calculate sample size
Common Approaches required for the analysis are as follows:-
- Descriptive statistics: t-tests and ANOVA for group comparisons.
- Correlation and regression of variable relationships
- Chi-square test for categorical relationships.
- Factor analysis of underlying constructs
Effective software tools for statistical analysis of survey data include SPSS, which has a user-friendly interface and comprehensive data management and analysis tools; AMOS, a powerful tool for structural equation modelling (SEM); and PLS-SEM, a subset of SEM that handles complex models and smaller sample sizes. Other alternatives include R, which is free and open-source software, and Python, a sophisticated programming language with data analysis packages. The decision is based on the researcher’s competence, analysis difficulty, and accessible resources.
To properly present survey findings visually, researchers should:
- Use relevant chart types based on the data (bar charts, histograms, pie charts, and line graphs).
- Ensure proper labeling of titles, axes, legends, and units.
- Select proper color schemes that promote comprehension.
- Use well-organized tables with clear headings and formatting to highlight crucial findings.
Selecting suitable visuals, employing clear labeling and formatting, and strategically using tables will help convey survey findings to readers effectively.
Data cleansing is an important step before evaluating survey data to ensure accurate and reliable results. The process consists of numerous methods and steps. First, researchers should look for missing data and then decide how to manage it, whether by removing incomplete cases or imputing values based on the previous responses. Post that, outliers have to be addressed. If they are in extreme quantities that the results can be distorted. Outliers can be identified using z-scores, box plots, or the Mahalanobis distance, and researchers must decide if they are true uncommon numbers or errors. Inconsistent responses should be deleted, or investigated and resolved. Researchers may also need to recode variables, such as converting string responses to numerical values, as well as construct composite scores or new variables based on their study objectives. Throughout the data cleaning process, it is essential to document all steps taken to ensure transparency and reproducibility of the analysis.
Determine the relevant statistical tests for your research by considering your research questions, hypotheses, and variable types. Use t-tests to compare means between two groups, ANOVA for three or more groups, and two-way ANOVA to investigate the relationship between a categorical independent variable and a continuous dependent variable. Correlation (Pearson’s r or Spearman’s rank) is used to study associations between continuous variables, whereas regression analysis (simple linear or multivariate) is used to forecast outcomes based on predictor variables. But before that, you should ensure that your data fits the test’s required assumptions, such as normality, variance homogeneity, and observation independence.
Several essential aspects go into determining the appropriate sample size for your survey: the intended degree of confidence (usually expressed as a percentage, such as 95%), the margin of error (how much variation you can tolerate in your results), and the population size you are studying. Generally, larger populations require larger sample sizes to ensure representativeness. Statistical formulas, such as those based on the normal distribution or t-distribution, help determine the minimum sample size required for the survey data. Online calculators and statistical tools make this procedure easier by combining these variables into a single calculation, guaranteeing that your survey results are statistically trustworthy and accurate.
Survey data are important for testing hypotheses and answering research questions in several ways. First of all, this data provide actual evidence that can either support or disprove the proposed hypotheses. By evaluating survey replies, researchers can uncover patterns, correlations, and trends in the data that are relevant to their study objectives. Moreover, surveys allow for the measurement of variables and constructs of interest, providing quantitative evidence to substantiate theoretical claims. Additionally, survey findings can guide further investigations or refine hypotheses based on unexpected or inconclusive results. Overall, survey data offer a systematic approach to gather and analyze data, enabling researchers to draw meaningful conclusions and contribute to advancing knowledge in their respective field.
When assessing survey data, it is important to conduct reliability and validity tests to ensure that the findings are of high quality. Reliability tests evaluate the survey instrument’s consistency and stability throughout multiple administrations, suggesting if the results can be believed to be consistent over time. On the other hand, validity tests, determine whether the survey measures what it claims to measure, ensuring that the data-driven findings are correct and useful. By carrying out these tests, researchers may confidently evaluate survey results, make educated decisions, and draw reliable conclusions for their study goals.