A Ph.D. in data analytics can lead to a multitude of options in a sector that is essential to the development of technology and the making of decisions in many different businesses. Analyzing datasets to make inferences about the information they hold, sometimes with the use of specialized hardware and software, is known as data analytics. As data has increased in volume, velocity, and variety in the digital age, data analytics has become increasingly important and is now a fundamental component of modern business and science (Brown & Davenport, 2020).
Those with a Ph.D. in data analytics are better able to address complicated challenges because they have the advanced knowledge and research abilities necessary to create new approaches, innovate analytical techniques, and use their expertise. Because data analytics is an interdisciplinary field, graduates can use their abilities in a variety of industries, including healthcare, finance, marketing, government, and academia (Provost & Fawcett, 2013).
Scope of a Ph.D. in Data Analytics
The scope of a Ph.D. in Data Analytics is extensive, covering various aspects such as Advanced Statistical Methods, Machine Learning and Artificial Intelligence, Big Data Technologies, Data Visualization and Ethics and Data Privacy. Each of these aspects contributes to a comprehensive education in data analytics, preparing graduates to tackle complex problems and drive advancements in their respective fields. For e.g.
Advanced Statistical Methods: Equip, students the ability to examine complicated datasets using advanced statistical approaches, enabling accurate and perceptive data interpretation (James, Witten, Hastie, & Tibshirani, 2013).
Machine Learning and Artificial Intelligence: Focuses on creating algorithms that can learn from data, anticipate the future, and handle decision-making tasks automatically, promoting innovation across a range of sectors (Hastie, Tibshirani, & Friedman, 2009)..
Big Data Technologies: involves being aware of and adept at using frameworks and tools made to efficiently handle, process, and analyse large amounts of data a critical ability in today’s data-driven environment (Chen, Mao, & Liu, 2014).
Data Visualization: Teaches the skill of graphical or pictorial data presentation, which aids in effectively and clearly conveying ideas to audiences that are both technical and non-technical (Rouse, 2019).
Ethics and Data Privacy: highlights the significance of carrying out moral research and protecting data security and privacy to promote integrity and confidence in data analytics methods (Montgomery & Runger, 2018).
Importance of Pursuing a Ph.D. in Data Analytics
The substantial influence a Ph.D. in data analytics has on one’s own professional development as well as on more general industry improvements makes it an extremely valued pursuit. First and foremost, Ph.D. candidates do creative research that strains the limits of existing understanding, producing novel findings and cutting-edge innovations that have the potential to completely transform a range of industries (Provost & Fawcett, 2013). The need for expertise gained from a Ph.D. degree is rising since businesses are depending more and more on data-driven decision-making processes. For those with advanced analytical skills, this strong demand results into a wealth of job options and competitive compensation (James, Witten, Hastie, & Tibshirani, 2013). Furthermore, Ph.D. holders can find employment in a variety of industries, including healthcare, finance, marketing, government, and academia, due to the multidisciplinary nature of data analytics (Hastie, Tibshirani, & Friedman, 2009). As a Ph.D. offers a profound degree of competence that is necessary for top-tier employment in both industry and academia, leadership opportunities are also abundant (Montgomery & Runger, 2018). Finally, Ph.D. holders can demonstrate the significant societal impact of their work by using data analytics to solve real-world issues. Examples of these contributions include bettering business efficiencies, healthcare outcomes, and public policy. (Chen, Mao, & Liu, 2014).
Potential Career Paths
Graduates with a Ph.D. in data analytics have a wide array of career paths available to them, including, academic researchers, data scientists, machine learning engineers, data engineers, business intelligence analysts, quantitative analysts, consultants, government analysts and many more. These roles demonstrate the versatility and demand for data analytics expertise across various sectors, offering Ph.D. graduates the opportunity to significantly impact a wide range of industries and societal issues.
Research Opportunities
Data analytics PhD students can investigate a range of cutting-edge research topics, including building systems for natural language processing (Provost & Fawcett, 2013) and predictive analytics (James et al., 2013), which builds models to forecast future trends based on historical data. They can research sophisticated neural networks and their uses in deep learning (Hastie et al., 2009), enhance big data infrastructure frameworks and tools (Chen et al., 2014), and use data in healthcare analytics (Montgomery & Runger, 2018) to enhance patient outcomes and healthcare efficiency. They can also read Social Network Analysis (Rouse, 2019) to learn about the dynamics and structure of social networks, and Ethics in AI (Brown & Davenport, 2020) to explore the moral implications of data analytics and artificial intelligence. These study topics highlight the depth and breadth of the field and provide Ph.D. candidates with the opportunity to make important contributions to a range of fields.
Conclusion
Obtaining a Ph.D. in Data Analytics provides a strong basis for pursuing a career in a quickly changing profession that is essential to several elements of contemporary society. Data analytics is an interdisciplinary field that enables graduates to utilize their abilities in various areas such as healthcare, banking, marketing, government, and academia. This wide range of industries ensures that there are diverse employment prospects available to them. The rising reliance of enterprises on data-driven decision-making to maintain competitiveness and foster innovation highlights the significance of this degree, given the increased demand for advanced analytical abilities.
Ph.D. candidates in Data Analytics acquire extensive theoretical understanding and practical abilities in managing intricate datasets, employing advanced analytical tools, and implementing state-of-the-art technology. Their blend of theoretical and practical knowledge allows them to make substantial contributions to their respective domains by conducting creative research and developing new approaches. Through the resolution of practical challenges, they propel progress that can result in greater healthcare results, streamlined company procedures, improved public policy, and several other benefits.
Furthermore, Ph.D. holders have the ability to occupy leadership and expert positions, which empowers them to have a significant impact on strategic decision-making and facilitate organizational transformation. Due to their proficiency in analyzing intricate data and producing practical insights, they are very important assets in any field. Ph.D. graduates in Data Analytics make valuable contributions that go beyond their immediate professional settings, as their work frequently tackles worldwide concerns and contributes to social advancement.
Essentially, undertaking a Ph.D. in Data Analytics provides students with the expertise and understanding to not only thrive in various professional fields but also to make significant and influential contributions to research, industry, and society as a whole. This program equips graduates to be leaders in data-driven innovation, playing a crucial role in creating the future through advanced analytics and well-informed decision-making.
References
- Brown, M., & Davenport, T. H. (2020). Big data at work: Dispelling the myths, uncovering the opportunities. Harvard Business Review Press.
- Provost, F., & Fawcett, T. (2013). Data Science for Business: What you need to know about data mining and data-analytic thinking. O’Reilly Media.
- James, G., Witten, D., Hastie, T., & Tibshirani, R. (2013). An Introduction to Statistical Learning with Applications in R. Springer.
- Hastie, T., Tibshirani, R., & Friedman, J. (2009). The Elements of Statistical Learning: Data Mining, Inference, and Prediction. Springer.
- Montgomery, D. C., & Runger, G. C. (2018). Applied Statistics and Probability for Engineers. Wiley.
- Chen, M., Mao, S., & Liu, Y. (2014). Big data: A survey. Mobile Networks and Applications, 19(2), 171-209.
- Rouse, W. B. (2019). Modeling and visualization of complex systems and enterprises: Explorations of physical, human, economic, and social phenomena. Wiley.
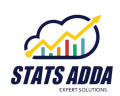